Table Of Content
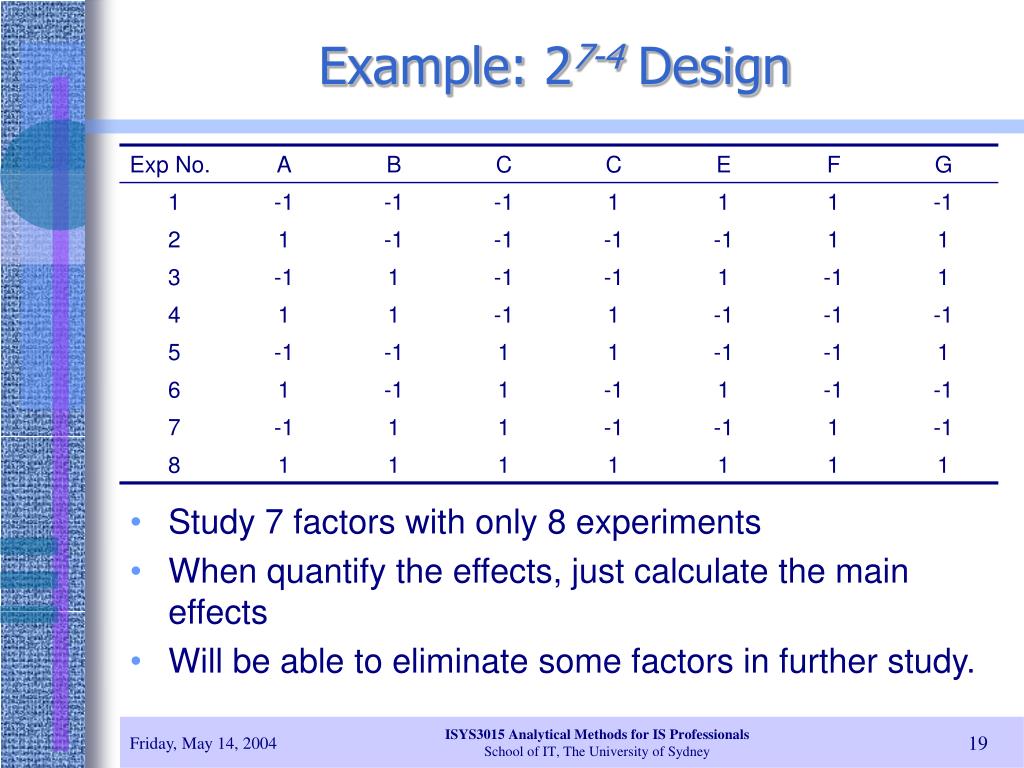
The choice of control conditions can also affect burden and complexity for both staff and patients. In this regard, “off” conditions (connoting a no-treatment control condition as one level of a factor) have certain advantages. They are relatively easy to implement, they do not add burden to the participants, and they should maximize sensitivity to experimental effects (versus a low-treatment control). Of course, less intensive (versus no-treatment) control conditions might be used for substantive reasons or because they ensure that every participant gets at least some treatment. For instance, RCTs need not have a true placebo or control condition. Thus, two different active treatments might be contrasted with one another in a two-group design, such as a comparison of two different counseling approaches (e.g., skill training vs. supportive counseling), each paired with the same medication.
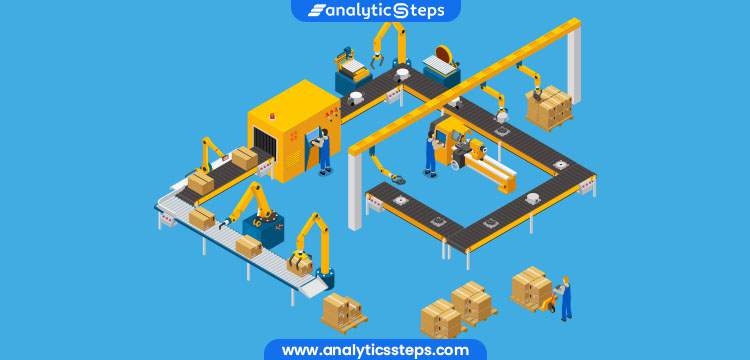
Advantages and disadvantages of factorial experiments
Minitab 15 Statistical Software is a powerful statistics program capable of performing regressions, ANOVA, control charts, DOE, and much more. Minitab is especially useful for creating and analyzing the results for DOE studies. It is possible to create factorial, response surface, mixture, and taguchi method DOEs in Minitab. Ignoring the first row, look in the last stage and find the variable that has the largest relative number, then that row indicates the MAIN TOTAL EFFECT.
Optimization of ICP-MS internal standardization for 26 elements by factorial design experiment - ScienceDirect.com
Optimization of ICP-MS internal standardization for 26 elements by factorial design experiment.
Posted: Sun, 23 Apr 2023 08:02:46 GMT [source]
3.10. Interpreting main effects and interactions¶
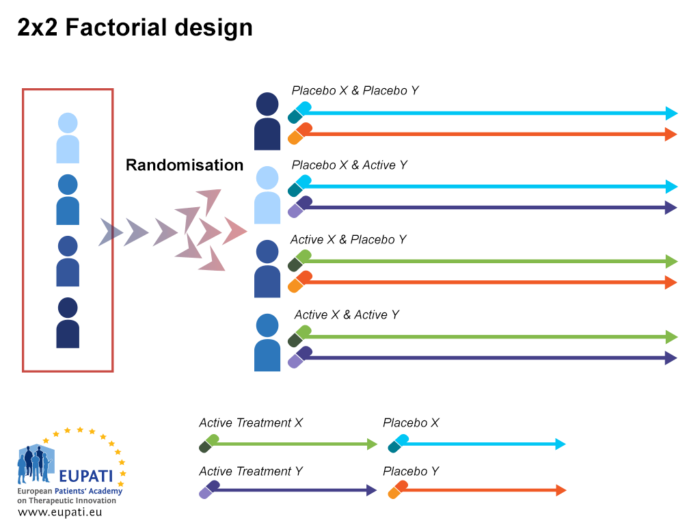
One example of a crossover interaction comes from a study by Kathy Gilliland on the effect of caffeine on the verbal test scores of introverts and extraverts [Gil80]. Introverts perform better than extraverts when they have not ingested any caffeine. But extraverts perform better than introverts when they have ingested 4 mg of caffeine per kilogram of body weight.
Types of Factorial Designs
It has been argued that factorial designs epitomize the true beginning of modern behavioral research and have caused a significant paradigm shift in the way social scientists conceptualize their research questions and produce objective outcomes (Kerlinger & Lee, 2000). Factorial design can be categorized as an experimental methodology which goes beyond common single-variable experimentation. In the past, social scientists had been transfixed on singular independent variable experiments and foreshadowed the importance of extraneous variables which are able to attenuate or diminish research findings. With widespread adoption of factorial design, social scientists could now... In statistics, a full factorial experiment is an experiment whose design consists of two or more factors, each with discrete possible values or "levels", and whose experimental units take on all possible combinations of these levels across all such factors.
Assigning Participants to Conditions
Although 2-level factorial designs are unable to explore fully a wide region in the factor space, they provide useful information for relatively few runs per factor. Because 2-level factorial designs can identify major trends, you can use them to provide direction for additional experimentation. For example, when you need to explore a region where you believe optimal settings may exist, you can augment a factorial design to form a central composite design. There are many simple examples of two independent variables being dependent on one another to produce an outcome.
How is this related to Factorial Designs?
In addition, the efficiency of a factorial experiment depends in part on the extent to which higher order interactions are not found. If interactions are found, and inferential statistics must be used to unpackage such interactions, such simple effects tests would require examining the effects of ICs in only subgroups of the sample. In essence, if it is necessary to follow-up an interaction by identifying which particular subgroups differ from one another, some of the efficiency of the factorial design may be lost.
5.2. Assessing Relationships Among Multiple Variables¶
Finally, including numerous ICs in an experiment could cause staff or counselors to spontaneously adjust their delivery of an intervention component because of their awareness of the total intensity of treatment provided to a participant. Counselors could either reduce the intensity of an intervention component when it is one of many that a participant receives, or they could increase the intensity of an intervention component if the participant is receiving little other treatment. In short, maintaining treatment delivery fidelity may take more care, training and supervision in a factorial experiment than in an RCT. When researchers study relationships among a large number of conceptually similar variables, they often use a complex statistical technique called factor analysis. In essence, factor analysis organizes the variables into a smaller number of clusters, such that they are strongly correlated within each cluster but weakly correlated between clusters.
If the number of combinations in a full factorial design is too high to be logistically feasible, a fractional factorial design may be done, in which some of the possible combinations (usually at least half) are omitted. The number of runs necessary for a 2-level full factorial design is 2k where k is the number of factors. As the number of factors in a 2-level factorial design increases, the number of runs necessary to do a full factorial design increases quickly. For example, a 2-level full factorial design with 6 factors requires 64 runs; a design with 9 factors requires 512 runs. A half-fraction, fractional factorial design would require only half of those runs.
Book traversal links for Lesson 5: Introduction to Factorial Designs
In addition, SuperGym offers 4 different workout plans, A through D, none of which are directly catered to any of the different types. Create an experimental factorial design that could be used to test the effects of the different workout plans on the different types of people at the gym. These equations can be used as a predictive model to determine wt% methanol in biodiesel and number of theoretical stages achieved at different operating conditions without actually performing the experiments. However, the limits of the model should be tested before the model is used to predict responses at many different operating conditions. In the previous section, we looked at a qualitative approach to determining the effects of different factors using factorial design.
Investigators may wish to adjust ICs to enhance their compatibility with other components. For instance, investigators might choose to reduce the burden of an IC by cutting sessions or contact times. This might reduce the meaning of the factor because it might make the IC unnecessarily ineffective or unrepresentative. From this table, we can see that there is positive correlation for factors A and C, meaning that more sleep and more studying leads to a better test grade in the class. Factor B, however, has a negative effect, which means that spending time with your significant other leads to a worse test score. The lesson here, therefore, is to spend more time sleeping and studying, and less time with your boyfriend or girlfriend.
To briefly add to the confusion, or perhaps to illustrate why these two concepts can be confusing, we will look at the eight possible outcomes that could occur in a 2x2 factorial experiment. We have usually no knowledge that any one factor will exert its effects independently of all others that can be varied, or that its effects are particularly simply related to variations in these other factors. From this one can see that there is an interaction effect since the lines cross. One cannot discuss the results without speaking about both the type of fertilizer and the amount of water used. Using fertilizer A and 500 mL of water resulted in the largest plant, while fertilizer A and 350 mL gave the smallest plant.
This is done to confirm that the independent variable was, in fact, successfully manipulated. For example, Schnall and her colleagues had their participants rate their level of disgust to be sure that those in the messy room actually felt more disgusted than those in the clean room. If an investigator anticipates severe problems from including a particular factor in an experiment, perhaps due to its nature or the burden entailed, s/he should certainly consider dropping it as an experimental factor. Indeed, the MOST approach to the use of factorial designs holds that such designs be used to decompose a set of compatible ICs, ones that might all fit well in an integrated treatment package (to identify those that are most promising). That is, one should include only those ICs that are thought to be compatible, not competitive.
Whereas, in the other conditions, there are only small increases in being hangry. An advantage of these graphs is that they display means in all four conditions of the design. Someone looking at this graph alone would have to guesstimate the main effects. If we made a separate graph for the main effect of shoes we should see a difference of 1 inch between conditions. Similarly, if we made a separate graph for the main effect of hats then we should see a difference of 6 between conditions.
In general, if the major goal of a study is to contrast directly one “treatment” with another treatment (e.g., a control treatment), then an RCT is usually the best choice. Note that here “treatment” is used to connote a set of intervention components (ICs); e.g., a particular type, dose, and duration of medication, type of counseling that is delivered for a particular number of sessions of a particular duration, and so on. The statistical analyses would reveal whether the experimental treatment “package” differs in effects from the usual care treatment. However, conducting an RCT that comprises ICs whose joint effects are unknown, poses clear risks.
We can look at this two ways, and either way shows the presence of the very same interaction. First, does the effect of being tired depend on the levels of the time since last meal? Look first at the effect of being tired only for the “1 hour condition”. We see the red bar (tired) is 1 unit lower than the green bar (not tired). So, there is an effect of 1 unit of being tired in the 1 hour condition. Next, look at the effect of being tired only for the “5 hour” condition.
No comments:
Post a Comment