Table Of Content
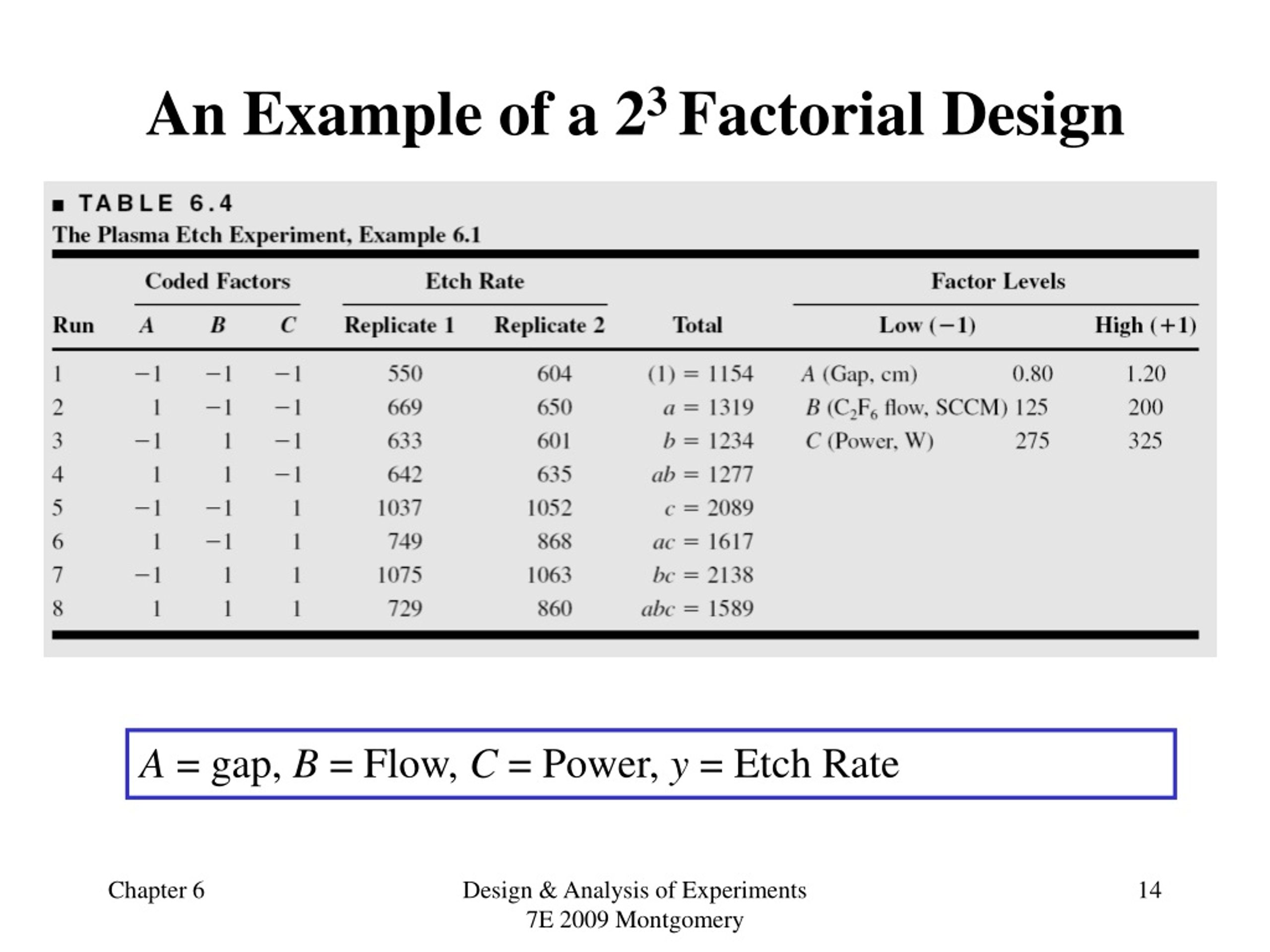
For example, you would be able to notice that all of these graphs and tables show evidence for two main effects and one interaction. Certainly any research evaluation of intervention effectiveness can pose analytic and interpretive challenges. However, some challenges are of particular relevance to factorial designs.
Factorial design-assisted supercritical carbon-dioxide extraction of cytotoxic active principles from Carica papaya leaf ... - Nature.com
Factorial design-assisted supercritical carbon-dioxide extraction of cytotoxic active principles from Carica papaya leaf ....
Posted: Fri, 08 Feb 2019 08:00:00 GMT [source]
How is this related to Factorial Designs?
However, it is important to note that interaction effects can be highly informative without simple effects tests (Baker et al., 2016; Box et al., 2005). In an RCT an “active” treatment arm or condition is statistically contrasted with a “control” treatment arm or condition (Friedman, Furberg, & Demets, 2010). The two conditions should be identical except that the control condition lacks one or more ICs or features that are provided to the active condition.
1.4. Measures of the Same Construct¶
We have done the hard work of finding an effect of interest, in this case the distraction effect. We think this distraction effect actually measures something about your ability to pay attention. If we think that we found an effect of distraction, maybe we could find a way to reduce distraction to help students focus on their homework?
Setting Up a Factorial Experiment
Like Pareto plots, Half Normal plots show which factors have significant effects on the responses. The factors that have significant effects are shown in red and the ones without significant effects are shown in black. The further a factor is from the blue line, the more significant effect it has on the corresponding response.
3.5. Identifying main effects and interactions¶
Often a researcher wants to know how an independent variable affects several distinct dependent variables. For example, Schnall and her colleagues were interested in how feeling disgusted affects the harshness of people’s moral judgments, but they were also curious about how disgust affects other variables, such as people’s willingness to eat in a restaurant. As another example, researcher Susan Knasko was interested in how different odors affect people’s behavior [Kna92]. She conducted an experiment in which the independent variable was whether participants were tested in a room with no odor or in one scented with lemon, lavender, or dimethyl sulfide (which has a cabbage-like smell).
Non-Manipulated Independent Variables
New spectrofluorimetric analysis of empagliflozin in its tablets and human plasma using two level full factorial design - ScienceDirect.com
New spectrofluorimetric analysis of empagliflozin in its tablets and human plasma using two level full factorial design.
Posted: Fri, 27 Mar 2020 04:21:46 GMT [source]
Another term for this property of factorial designs is “fully-crossed”. Including additional factors in an experiment might certainly affect the significance tests of a given factor. The reduction in power will be greater if we control experiment-wise error, due to the larger number of main and interaction effects.
Note that this is not a new level to our Distraction IV, but a whole new IV. In one condition, people will get $5 for every difference they find (so they could leave the study with lots of money if they find lots of differences). In the other condition, people will get no money, but they will still have find differences (comparison control condition). Imagine that the results show that people found an average of four differences when they were distracted, and an average of 10 differences when they were not distracted. The effect of distraction is a mean of six differences found in the picture. It’s the difference between performance in the Distraction and No-Distraction conditions.
Onwards, the minus (−) and plus (+) signs will indicate whether the factor is run at a low or high level, respectively. Frank Yates made significant contributions, particularly in the analysis of designs, by the Yates analysis. This website is using a security service to protect itself from online attacks. There are several actions that could trigger this block including submitting a certain word or phrase, a SQL command or malformed data. As expected, we the average height is 6 inches taller when the subjects wear a hat vs. do not wear a hat. So, the main effect of wearing hats is to add 1 inch to a person’s height.
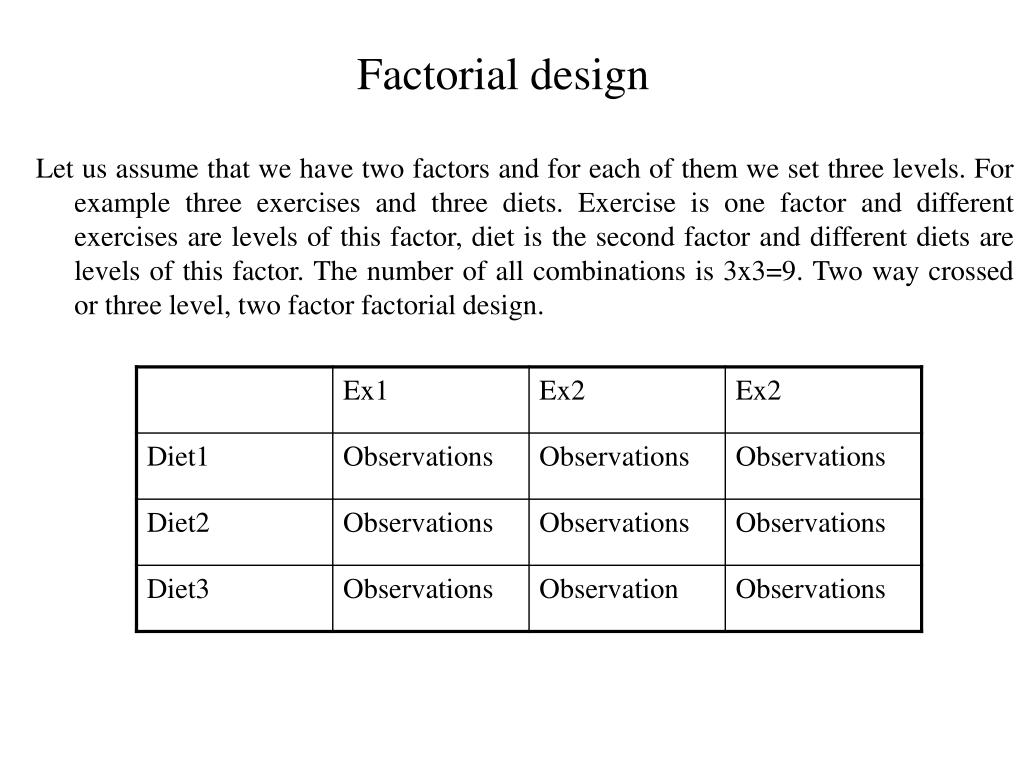
Modifying DOE Table
For example, an effect of participants’ moods on their willingness to have unprotected sex might be caused by any other variable that happens to be correlated with their moods. The simplest way to understand a main effect is to pretend that the other independent variables do not exist. If you do this, then you simply have a single-factor design, and you are asking whether that single factor caused change in the measurement. For a 2x2 experiment, you do this twice, once for each independent variable. In the table, a yes means that there was statistically significant difference for one of the main effects or interaction, and a no means that there was not a statisically significant difference. As you can see, just by adding one more independent variable, the number of possible outcomes quickly become more complicated.
As with any statistical experiment, the experimental runs in a factorial experiment should be randomized to reduce the impact that bias could have on the experimental results. In the 2 × 3 example, for instance, the pattern of the A column follows the pattern of the levels of factor A, indicated by the first component of each cell. Regardless of whether the design is between subjects, within subjects, or mixed, the actual assignment of participants to conditions or orders of conditions is typically done randomly.
The next step is selecting which terms will be analyzed for the responses. Explore Psychology provides information about psychology and mental health. The material found on this site is for informational purposes only and is not intended to be used for diagnosis or treatment purposes. So, the researchers decided to look at how the amount of sleep and caffeine influence test performance.
When conducting an experiment, varying the levels of all factors at the same time instead of one at a time lets you study the interactions between the factors. The primary way of doing this is through the statistical control of potential third variables. Instead of controlling these variables by random assignment or by holding them constant as in an experiment, the researcher measures them and includes them in the statistical analysis. Consider some research by Paul Piff and his colleagues, who hypothesized that being lower in socioeconomic status (SES) causes people to be more generous [PKCote+10]. They measured their participants’ SES and had them play the “dictator game.” They told participants that each would be paired with another participant in a different room.
2 x 2, 3 x 3, and 2 x 3 designs all have two numbers in the notation and therefore all have two independent variables. The numerical value of each of the numbers represents the number of levels of each independent variable. A 2 means that the independent variable has two levels, a 3 means that the independent variable has three levels, a 4 means it has four levels, etc.
This would mean that each participant would be tested in one and only one condition. In a within-subjects factorial design, all of the independent variables are manipulated within subjects. All participants could be tested both while using a cell phone and while not using a cell phone and both during the day and during the night. This would mean that each participant would need to be tested in all four conditions. The advantages and disadvantages of these two approaches are the same as those discussed in Chapter 5. The between-subjects design is conceptually simpler, avoids order/carryover effects, and minimizes the time and effort of each participant.
No comments:
Post a Comment